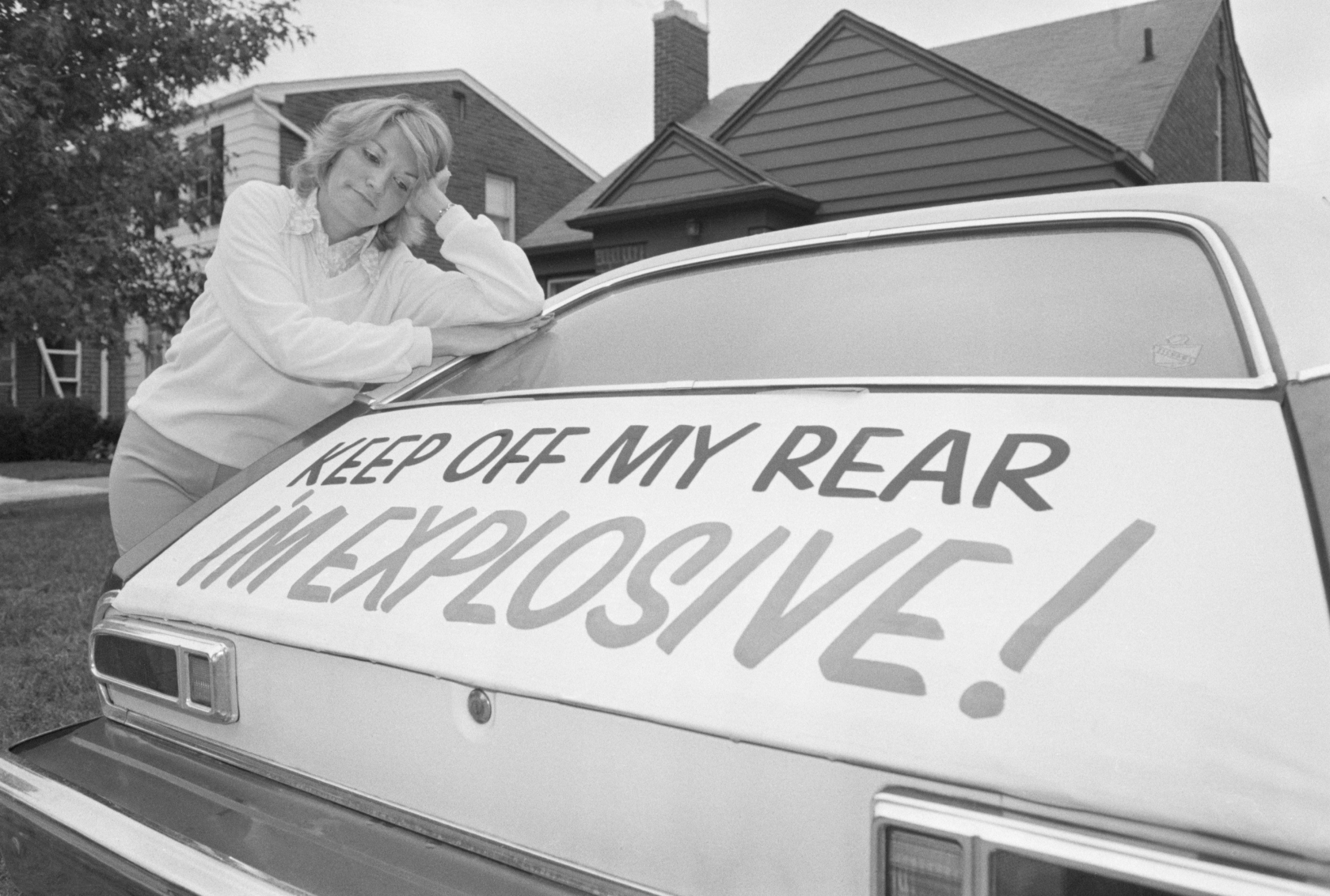
(Original Caption) GROSSE POINT FARMS, MICH.: Patty Ramge appears dejected as she looks at her Ford Pinto where she put a sign on the rear of the automobile stating “Keep off my rear, I’m explosive.” Mrs. Ramge put the sign because of the firey accidents involving Pintos whose gas tanks exploded after being hit from behind killing or seriously injuring the occupants.
In the late spring of 1972, Lily Gray was driving her new Ford Pinto on a freeway in Los Angeles, and her thirteen-year-old neighbor, Richard Grimshaw, was in the passenger seat. The car stalled and was struck from behind at around 30 mph. The Pinto burst into flames, killing Gray and seriously injuring Grimshaw. He suffered permanent and disfiguring burns to his face and body, lost several fingers and required multiple surgeries.
Six years later, in Indiana, three teenaged girls died in a Ford Pinto that had been rammed from behind by a van. The body of the car reportedly collapsed “like an accordion,” trapping them inside. The fuel tank ruptured and ignited into a fireball.
Both incidents were the subject of legal proceedings, which now bookend the history of one of the greatest scandals in American consumer history. The claim, made in these cases and most famously in an exposé in Mother Jones by Mike Dowie in 1977, was that Ford had shown a callous recklessness for the lives of its customers. The weakness in the design of the Pinto — which made it susceptible to fuel leaks and hence fires — was known to the company. So too were the potential solutions to the problem. This included a number of possible design alterations, one of which was the insertion of a plastic buffer between the bumper and the fuel tank that would have cost around a dollar. For a variety of reasons, related to costs and the absence of rigorous safety regulations, Ford mass-produced the Pinto without the buffer.
Most galling, Dowie documented through internal memos how at one point the company prepared a cost-benefit analysis of the design process. Burn injuries and burn deaths were assigned a price ($67,000 and $200,000 respectively), and these prices were measured against the costs of implementing various options that could have improved the safety of the Pinto. It turned out to be a monumental miscalculation, but, that aside, the morality of this approach was what captured the public’s attention. “Ford knows the Pinto is a firetrap,” Dowie wrote, “yet it has paid out millions to settle damage suits out of court, and it is prepared to spend millions more lobbying against safety standards.”
It is hard to imagine these days, but half a century ago car crashes were generally blamed entirely on the driver, despite cars incorporating very few safety standards into their manufacture. Every problem was attributed to the responsibility of the person behind the wheel. The automotive industry lobbied hard to limit its responsibility for deaths on the road and treated safety as incompatible with selling cars. “Self-styled experts with radical and ill-conceived proposals,” warned John F. Gordon in 1961, “[think] the only practical route to greater safety [is] federal regulation of vehicle design.” Gordon was the president of General Motors, and he was speaking to the National Safety Congress. His skepticism was undisguised: “The suggestion that we abandon hope of teaching drivers to avoid traffic accidents and concentrate on designing cars that will make collisions harmless is a perplexing combination of defeatism and wishful thinking.” His address was met with enthusiastic applause. The powerful Business Council backed the industry, as did many other leaders of American capitalism. W. B. Murphy, president of the Campbell Soup Company, was openly disdainful: “It’s of the same order of the hula hoop,” he said of the concern about car safety. ‘Six months from now we will probably be on another kick.”
This attitude was, in part, a product of the lax regulatory environment. The national regulator was understaffed and underfunded. Under the Nixon presidency, key positions in agencies remained unfilled. In the late 1960s, Dr. Thomas Malone, chairman of the National Motor Vehicle Safety Advisory Council, wrote to the secretary of the National Highway Traffic Safety Administration about the problem of underfunding: “The Federal funding is not commensurate with the size of the problem. The serious gap between authorized and appropriated funding has handicapped the forward motion of the program.” But the political establishment was not interested in applying much pressure to one of the biggest industries fueling the postwar boom in America. It was easier to adopt the line that road safety was a matter of individual responsibility than to tackle the industry head-on.
For these reasons, people continued to die — despite the known technological possibilities for making cars and roads safer. The National Academy of Sciences described the dangers of car travel in 1966 as “a neglected epidemic of modern society” and “the nation’s most important environmental health problem.” It is not certain how many people lost their lives or were injured as a result of Pinto fuel tanks igniting: estimates vary from hundreds to thousands. But the scandal acted as a lightning rod, prompting regulators to rethink the accepted wisdom touted by the industry and consider whether the requirements they imposed on manufacturers were sufficient.
The accounting system used by Ford engineers to weigh death and serious injury against cost and marketability was callous and impossible to justify. This was a preventable disaster. It was also bigger than any single company. In the 1960s, GM’s Corvair had similar design problems that affected the car’s steering and resulted in over a hundred lawsuits. The tragedy did not begin with the manufacturing of the car or even when it failed in testing. The tragedy, according to lawyer and consumer activist Ralph Nader, “began with the conception and development of the Corvair by leading GM engineers.” It was an industry-wide culture that failed to consider the impact of design on the end user, that deferred and outsourced moral responsibility to consumers. Just like the Pinto, the Corvair was a problem by and of design.
This is not an attempt to pin blame on evil engineers or designers. The people who made these cars were working in a specific corporate climate. Their organizations were led by ruthless executives. The leadership of companies like Ford and GM ignored safety concerns in competition with other companies that did the same. It was not even a problem confined to the auto industry; there have been many other similar scandals involving corporate indifference to the human consequences of poorly designed consumer products. These scandals are not aberrant; they occur in a context, and to avoid them happening again requires a political strategy to attack the logic that produces them.
*
Professor Latanya Sweeney of Harvard University typed her name into Google; she was searching quickly for an old paper she had written. She was shocked to see an ad pop up with the headline “Latanya Sweeney–Arrested?” Sweeney does not have a criminal record. She clicked on the ad link and was taken to a company website selling access to public records. She paid the sum to access the material, which confirmed she had no criminal history. When her colleague Adam Tanner did a similar search, the same ad for a public records search company appeared, but without the inflammatory headline. Tanner is white; Sweeney is African American.
Sweeney decided to study the placement of these ads to see if there was a pattern. She did not expect her results to be definitive. But her research produced a clear outcome: “Ads suggesting arrest tend to appear with names associated with blacks and neutral ads or no ads tend to appear with names associated with whites, regardless of whether the company has an arrest record associated with the name.”
In other words, a greater percentage of ads with the word “arrest” appeared in ad text for first names that are associated with black people than for “white” first names. The presence of an actual criminal record did not appear to be the deciding variable.
How did this come about? To explain requires some unpacking of the online ad business. Each time you click on a website, an instant auction for ad space takes place between companies competing for your attention. As we know, surveillance capitalism has all sorts of ways for determining how valuable you are to a marketer, to allow platforms to make accurate bids on your eyeball time. These companies know more about our habits than we do. They have a detailed picture of our abstract identity — a history of our sense of self, defined by and for consumption — and they are using this information to send us marketing messages at optimal moments. Left to their own devices, this creates a situation where new technologies reproduce real-world forms of oppression.
The options around ad spaces are tailored in more ways than one. Google allows companies to tailor not just which audience sees the ad but also the content of the ad itself. As Sweeney explains:
Google understands that an advertiser may not know which ad copy will work best, so an advertiser may give multiple templates for the same search string and the “Google algorithm” learns over time which ad text gets the most clicks from viewers of the ad. It does this by assigning weights (or probabilities) based on the click history of each ad copy. At first all possible ad copies are weighted the same, they are all equally likely to produce a click. Over time, as people tend to click one version of ad text over others, the weights change, so the ad text getting the most clicks eventually displays more frequently. This approach aligns the financial interests of Google, as the ad deliverer, with the advertiser.
Because of the way the algorithm has been designed, the machine learns to associate African American names with criminality. Even if you personally do not click on the ad, you experience the consequences of the machine learning from what other users clicked, which restricts the choices displayed to all subsequent users.
One possible response to this is that the algorithm is neutral, that it is just a vehicle for the ad, automatically responding to how people use it; algorithms aren’t racist, people are racist. But the algorithm is built in a way that also confirms implicit biases that exist in the real world, and it does so over and over again. The assumption that African American people are less trustworthy than white people is a commonly held form of implicit bias. It has real-world implications in a whole range of ways, from the success of job applicants to the split-second decisions made by police when pointing their guns at people. In Sweeney’s study, we see this attitude reproduced in the world of digital technology, intentionally or otherwise. This is not a mystery or an unfathomable outcome. Google is not entirely responsible for racism having an impact on automated advertising, but it cannot shirk responsibility for it. Ford was not solely responsible for hundreds, possibly thousands of cases of people being burned alive in their Pintos, but the court of public opinion rightly felt that it could have easily prevented them if it had designed the car differently.
Part of the reason that this ad ended up being racist is because the process of designing algorithms and training them on real-world data takes place with basically zero transparency. The inputs are secret, and there is no formal regulation properly adapted to these processes. The bad experiences of users like Sweeney do not show up in the cost-benefit analyses for these companies when selling ad spaces. There isn’t even a formal way to complain about it. There is barely a way to know about it. Biased algorithms exert considerable and increasing influence over many aspects of our lives. So long as they remain hidden or unexamined, we are allowing all manner of dangerous and oppressive practices to become embedded in new technologies, as machines learn to absorb the implicit biases that exist in the real world.
The idea that none of this is Google’s responsibility loses sway when we consider that the power is in its hands to know and act on this information. Google decision makers knew the content the advertiser — their paying customer — plans to use. They are best placed to know the potential problem and how it might manifest, because they designed the system. At the moment, they calculate that the costs of finding and fixing these problems is higher than the cost of ignoring them, which is borne by others. We must find ways to change that calculus.
Google executives should bear the responsibility for the outcomes generated by their technology when it does what it is designed to do. In this case, Google is providing a service that is doing what it is designed to do: monetize advertising most effectively. In other words, the importation of racism into digital technology and the failure to consider implicit bias in design processes is not a bug, it is a feature of technology capitalism.
For this reason, what is perhaps most contemptible of all is that there is no actual reason for this kind of design discrimination to happen. The web is a space where oppressive attitudes could be structurally minimized, acknowledged and dismantled. Not only could we have policies in place that forestall racist ad placement, we could also engineer better representations of diversity that actively minimize prejudice. We could anticipate implicit bias and figure out ways to neutralize its effects in advance; we could prevent companies from capitalizing on its existence. We could design and build digital infrastructure that helps socialize people against discriminatory implicit biases. Such a prospect raises all sorts of interesting questions about how it might work in practice, and we could collectively start to grapple with this task.
We could campaign for and draft legal regulation of the design and engineering processes, much as consumer rights advocates demanded that the federal government implement safety regulations for cars. We need to establish rules that prioritize the goal of dismantling oppression over that of monetizing the web. This is a precious opportunity. If we simply wait for these problems to present themselves, or address them piecemeal as they emerge, we will miss the iceberg for the tip. We will allow an industry to entrench itself and marshal its forces against transparency and accountability while pinning blame on users when things go wrong. At present, we are reliant on people like Sweeney to discover that these problems exist — and she only did so by accident.
Data scientist Cathy O’Neil, in observing the combination of careless logic, lack of feedback, and substandard data inputs that characterizes many algorithms, calls them “weapons of math destruction.” She writes about how they have a tendency to “blithely generate their own reality.” The perceived neutrality of digital processes provides cover for the sloppy and divisive treatment of people, while outsourcing the management of a range of activities to insidious effect. “Managers assume that the scores are true enough to be useful, and the algorithm makes tough decisions easy,” she writes. “They can fire employees and cut costs and blame their decisions on an objective number, whether it’s accurate or not.” Computerized processes for determining answers to complex questions in the age of big data create exciting and transformative possibilities, but they also bestow on bad governance and management an undue gloss of accuracy and neutrality.
Through the small but disconcerting porthole opened by Sweeney, we glimpse a vast ocean of activity. Algorithms are being used in all sorts of ways that can have profound impacts on people. One example is reliance on automated processes for filtering job applicants, which can be biased against people with a history of mental illness or those for whom English is a second language. Another is standardized testing for college admission. Admissions processes, particularly if they do not require standardized tests, might use a predicted score as a proxy based on the applicant’s demographic characteristics, with no clear idea of the accuracy of that substitute.
Algorithms are also used for deciding on parole applications, which rely on forms filled out by caseworkers without any indication of how the responses impact the output of the algorithm. In a deeply offensive example, a Google photo application, which automatically sorts photos by subject matter, once labelled a photo of some black people as gorillas. Secretive, proprietary algorithms have a tendency to produce thinly veiled bias disguised as scientific logic. These problems are not mere blunders, much in the same way that the bad steering in Corvairs or lack of buffering for the Pinto fuel tanks were not simply unfortunate errors. They are symptomatic of a flawed design process.
These kinds of processes affect people in society differently. As O’Neil points out, machines are cheap and efficient; their decision making is more likely to be imposed upon poor people. “The privileged,” she observes, “are processed more by people, the masses by machines.” And it is almost impossible for people who confront these machines to question or challenge their decisions, if they even know that they are being made. Walmart, for example, has created catalogs for low-income audiences, which market a disproportionate amount of junk food relative to healthier options. Data about arrests can also be used in oppressive ways when cross-referenced with other data sets. Evidence of an arrest can mean that automated résumé-sorting software may pre-emptively exclude a candidate from consideration for jobs or deny one access to consumer finance, sometimes even after the arrest has been expunged from the public record. Machine learning is routinely used and tested on the poor, and it is the most vulnerable in society that end up dealing with the consequences.
There is undeniably a class dynamic to the impact of oppressive algorithms. Technology, especially under the stewardship of the elite, mirrors the value systems that underpin social divisions. Many of our current conversations about the dangers of artificial intelligence are dominated by the possibility that such technology will lead to a third world war. However valid, the framing of these concerns reveals something deeper. Many of the people driving these conversations are rich white men — and, as researcher Kate Crawford points out, “perhaps for them, the biggest threat is the rise of an artificially intelligent apex predator. But for those who already face marginalization or bias, the threats are here.”
There is a growing and sophisticated network of algorithms that generates all sorts of social, economic and cultural consequences. Abstract identification invariably relies on profiling based on data, or discrimination in relation to our abstract identities. This is a practice of data discrimination. That is, communities and individuals are segmented into audiences for marketing purposes, often on the basis of superficial assumptions about specific and incomplete data, which has highly divisive effects and accelerating impacts. As Adam Greenfield argues, “contemporary technologies never work as stand-alone, isolated, sovereign artifacts.” Networks collect and exchange data, which has been channeled in various directions as a result of path dependency, and this is amplified by market functions and social prejudice. The decision-making function of machines has the capacity to not just reproduce traditional social fault lines but also to exacerbate them.
*
By 1978, under pressure from advocates and the regulators, Ford agreed to voluntarily recall all Pintos built between 1971 and 1976. This decision came just months after a jury awarded $126 million in damages to Richard Grimshaw (the award was reduced by the trial judge but remained substantial). The decision in Grimshaw was affirmed on appeal, with the court noting that “the conduct of Ford’s management was reprehensible in the extreme.” It found that management “exhibited a conscious and callous disregard of public safety in order to maximize corporate profits … endangering the lives of thousands of Pinto purchasers.” Months later, criminal proceedings were brought against Ford for the deaths of the teenaged girls in Indiana. Ford was acquitted in this case. But it did end up settling subsequent claims against it made in relation to the Pinto. By 1980, the model was discontinued.
The Pinto scandal should not be seen as an aberration of amoral engineers who failed in the design process to properly value human life. While it is important for everyone involved in the design process to be thoughtful and aware about their work, it is also important to acknowledge that these engineers and designers were operating in a business-driven context. The executive leadership at Ford made critical decisions and ignored important information that was given to them. They also operated in a fiercely competitive market, where regulators were asleep at the wheel or, worse, captured by the industry. Changing this dynamic required the work of journalists, activists and lawyers. It also required new forms of regulation that anticipated the risk of dangerous design and created conditions in which engineers could proceed ethically without jeopardizing their employment. Under capitalism, it is an unceasing battle to defend human life and dignity from the magnetism of the bottom line.
Computer code itself functions as a form of law. It is written by humans and it regulates their behavior, like other systems of power distribution. It is not an objective process or force of nature. It expresses a power relation between coder and user, and it will reflect the system in which coders work. “Code is never found,” Lawrence Lessig reminds us. “It is only ever made, and only ever made by us.” Letting the free market determine these matters means that digital technology risks reproducing discrimination under the cover of an inscrutable process. Joy Buolamwini is a founder of the Algorithmic Justice League, which aims to publicize and challenge bias in algorithms: “We don’t have to bring the structural inequalities of the past into the future we create,” she argues. In her view, we can only achieve this if we organize around a specific purpose with intention.
Some legal prohibitions on discrimination already exist that would capture some of these examples as they manifest in biased code. But there are not enough such curbs, while enforcing them will require updated powers for regulators. Identifying these problems will also involve imposing greater duties on tech companies. We need to demand that lawmakers and public agencies, under color of democratic authority, intervene in these markets and both promulgate and enforce design requirements on the industry. “A democratic government is far better equipped to resolve competing interests and determine whatever is required (to improve transport safety) than are firms whose all-absorbing aim is higher and higher profits,” wrote Nader in 1965 of the car industry. The same is true of technology companies and government today.
Importantly, workers who build this technology also have a role to play in changing the culture of design. Ethical design considerations can serve as an industrial and political organizing tool, acting as a bulwark against predatory business practices. “Technological professionals are the first, and last, lines of defense against the misuse of technology,” writes Cherri M. Pancake, president of the world’s largest organization of computer scientists and engineers, the Association for Computing Machinery. In 2018, the organization published an updated code of ethics, which requires developers to identify possible harmful side effects or the potential for misuse of their work, consider the needs of a diverse set of users, and take special care to avoid the disenfranchisement of groups of people. Among the feedback received about the code by the ACM was this comment from a young programmer: “Now I know what to tell my boss if he asks me to do something like that again.” Resolving the ethical considerations involved in design is rarely a straightforward task, but it is not impossible, and creating space for technologists to consider the options and work through them fully is an important component of this.
We can start to see how this looks in practice already, as workers in major technology companies are taking on their bosses on ethical grounds. Microsoft employees organized to demand that their company cancel its contracts with Immigration and Customs Enforcement and other clients who directly enable them. “As the people who build the technologies that Microsoft profits from, we refuse to be complicit,” they wrote. “We are part of a growing movement, [comprising] many across the industry who recognize the grave responsibility that those creating powerful technology have to ensure what they build is used for good, and not for harm.” This ethical and ultimately political decision to refuse to build harmful technology is not made on an individual basis, but a collective, industrial one. Similar insurgencies are happening at Google, with 4,000 workers signing a petition opposing a project on behalf of the military and senior engineers refusing to work on specific projects that would enable the company to win sensitive military contracts. This kind of collective organizing has immense potential to transform the culture of technology production through self-organization, which opens up ethical questions much more effectively than any top-down form of discipline or compliance.
*
Design processes in the digital age need to make it easy for engineers to take more account of users’ interests. But how we come to understand users’ interests can be a complex question, and it will take time and effort to incorporate these interests into design processes. As we develop technology and explore its potential, we may also have to impose limits on our technological capabilities if we are to avoid creating harm.
This is a particularly important consideration as we witness the expansion of the Internet of Things — as more everyday objects are fitted with network connectivity. You can buy a fridge or oven or a home climate system that is connected to the Internet and can therefore transfer data between objects and people. The range of products under development (which often span from excessive to useless, it must be said), also reveals the potential positives of this kind of technology, such as the assistance it could offer to people with mobility problems in the home. Amazing progress is being made with technology built to help with various forms of disability. There is also the promise of convenience: if your smart suitcase fails to show up, you can go online and track it down.
But there is a troubling side to smart objects too. As we bring more devices into our home that communicate with outsiders in ways we cannot control, the Internet of Things is arguably turning into an enormous surveillance apparatus. This is a particular problem for the vulnerable. As Elise Thomas writes, on the topic of technology and domestic violence:
Advances in technology are both a blessing and a curse for the targets of domestic violence. New “smart” technology can make it easier for them to contact help and document the abuse — but equally, it can be misused to monitor their activities, eavesdrop on their conversations and even track their location in real time …
Once upon a time, a phone number could be enough to get someone killed — so what does it mean for the targets of domestic violence when we move into a world where it’s possible for someone with access to the right devices to track every move, hear every breath, read every heartbeat on a screen?
The implications of the Internet of Things for survivors of domestic violence are profound. As more devices become connected to the network without our ability to control these data flows, it becomes much easier for others to access large amounts of information about us. Wearable technology can be hacked, cars and phones can be tracked, and data from a thermostat can reveal whether someone is at home.
This depth and breadth of data is frightening for anyone who has experienced an abusive relationship — that is, for a lot of people. More than a third of women and more than a quarter of men in the United States have experienced rape, physical violence, or stalking by an intimate partner in their lifetime. Technological abuse is now standard practice among people who choose to use violence. In a 2014 survey of service providers for survivors of domestic violence, 97 percent report that their users experience harassment, monitoring, and threats by abusers through the misuse of technology. This is often harassment and abuse by phone, such as text messages and social media postings. But 60 percent of service providers also reported that abusers have spied or eavesdropped on the children and the survivor by means of technology. Abusers do this by giving gifts to the child or planting devices on the child’s belongings; 11 percent reported instances of toys with hidden “spying” technology. The survey also found that 45 percent of programs report instances of abusers trying to locate survivors through technology. These findings were supported by another piece of research that found that 85 percent of the shelters surveyed work with survivors whose abusers tracked them using GPS, and 75 percent work with survivors whose abusers eavesdropped on their conversation remotely using hidden smartphone apps. Nearly half of the shelters surveyed ban the use of Facebook because of fears about revealing locational information to stalkers.
These social problems are not the responsibility of tech companies to fix, but they are an undeniable feature of the society in which companies operate. They are something that ought to be considered in the early development stages and accommodated during the design process. We are often told how handy and futuristic it is to connect more and more personal devices to the Internet. But not everyone feels that way. The generation of huge quantities of personal data, and our inability to control how this data is collected and stored, has serious consequences, especially for certain groups. Yet the experiences of large sectors of society, particularly those that are vulnerable, commonly appear to be absent from the design process.
This approach ends up affecting everyone, not just those with specific vulnerabilities: as technology capitalism finds new ways to learn about our personal lives, we can expect the government’s spies to be finding their own way onto this information gravy train. In testimony submitted to the US Senate in February 2016, James Clapper, then director of National Intelligence, conveyed this very well. “In the future, intelligence services might use the [Internet of Things] for identification, surveillance, monitoring, location tracking, and targeting for recruitment, or to gain access to networks or user credentials,” Clapper said. The state often makes use of industry innovations to repurpose them for their own interests. Writer Evgeny Morozov put it succinctly: “In case you are wondering what ‘smart — as in ‘smart city’ or ‘smart home’-means: Surveillance Marketed As Revolutionary Technology.”
This indifference on the part of companies to the experiences of their users stems in part from a specific understanding of usability and utility. The automotive industry was in a similar mind-set when the Pinto scandal broke. In Unsafe at Any Speed, Ralph Nader points out that it was largely uninterested in spending money to improve safety. About $166 was spent on research for every traffic fatality, a quarter of which was from industry. By way of comparison, industry and government together spent $53,000 on safety work arising from each death in the aviation industry. While car companies were happy to invest in making cars faster or slicker, when safety features threatened aesthetic design principles, the industry resisted them. Technology companies, insistent on connecting everything to the Internet of Things and designing products with a certain kind of user in mind, occupy a similar paradigm. They like to talk about servicing customers, but this purpose is understood through a specific and narrow frame.
One of the most obvious reasons for this is that the people designing much of our digital technology are drawn from a specific demographic. The overrepresentation of white men in Silicon Valley is well known. According to Reveal, from the Center for Investigative Reporting, in 2016 ten large technology companies in Silicon Valley did not employ a single black woman. Three had no black employees at all and six did not have a single female executive.
Some companies are doing better than others, and many major companies release diversity reports, which is a change from years past. But white people, particularly men, remain overrepresented in the tech industry both relative to the population and compared to the private sector overall. This trend is even more extreme among executive leadership. It is for this reason that we see design approaches to networked devices that are indifferent to threats like domestic violence, despite the fact that it is a prevalent problem within the community. Given a specific cohort of people in the room, the decisions made by these companies will inevitably display particular biases, and there will be a lower chance of anyone thinking to correct for them.
There is a class dynamic to this also. Adam Greenfield points out that the Internet of Things is being designed by a group of people who have completely assimilated services like Uber, Airbnb and Venmo into their lives, despite the fact that this does not reflect a universal experience. They have embraced the digitalized, individualized, optimized and commodified version of the world: “These propositions become normal to them, and so become normalized for everyone else as well.” In reality, a significant number of people have never used these services or even heard of them. But they are not the constituency being serviced by technological development. One journalist observed that the 2018 Consumer Electronics Show seemed “less about real innovation breakthroughs solving unmet needs and more about incrementally improved nice-to-haves for the 1%.” Another commentator was more blunt: “[San Francisco] tech culture is focused on solving one problem: What is my mother no longer doing for me?” Too often, the people involved in the design process come from an experience of affluence (as well as a particular gender), and this affects the development of technology more generally, with repercussions for us all.
Diversity within the ranks of programmers is vital to changing this culture. This is not just a pipeline problem but also a problem within technology companies that will require changing things like recruitment practices, accountability processes, and policies relating to working conditions. The Google walkout of 2018 involved 20,000 employees of the company stopping work to protest how the company manages sexual misconduct cases and highlight the implications for women in the workplace. The workers won some of their demands almost immediately, but there is more to be done. It serves as an inspiring example of the sophisticated barriers that exist to cultivating a diverse workforce and how they might be dismantled through organizing. Tech companies ignore this at their peril. The lack of diversity in the industry is now so glaring a problem that calls for change, and proposals for achieving it, attract widespread and mainstream attention. While the prospects of success in this respect are an interesting topic for reflection, they are already the focus of considerable discussion and activity. I want to turn to a broader question.
Changing the representation of diverse groups within the maker class is not enough. We need to change the culture around ethical design. Executives who urge their programmers to move fast and break things clearly expect someone else to pick up the pieces. We need to argue that the paradigm should instead be about building thoughtful programs that are respectful of the impact of design and thinking critically about the identity of the user. Ethical quandaries should not be considered above the pay grade of the programmer, they ought not to be mentally outsourced to others — but that requires programmers to have the skills and the capacity to navigate them. This will mean expanding existing educational programs on ethics and making them more mainstream. But to put such programs into practice, tech companies must also provide the space for accommodating these deliberative processes. That may also mean prioritizing human involvement in decision-making and moderation over automated processes, even when it is more costly and less efficient.
Creating a programming culture that places greater value on the importance of empowering people and limiting the risk of harm is a necessary (if insufficient) step to addressing some of these problems. Over time, such work might broaden into questions about political power and ultimately foster a culture that celebrates technology for peaceful purposes and challenges its prevalence in industries of violence and oppression, such as prisons, policing and the military.
People buy and use the available products voluntarily, of course, and we respect their right to make these choices. But it is impossible to think that every one of these people fully understands the nature and implications of recent technology. Samsung caused controversy when it included in its Smart TV privacy policy a warning to customers that they should “be aware that if your spoken words include personal or other sensitive information, that information will be among the data captured and transmitted to a third party through your use of Voice Recognition.” Even a Barbie is not safe from Internet connectivity: Mattel released a version of the doll that uses Wi-Fi to send data back to the company for research and development. But she comes with her own vulnerabilities. Security researcher Matt Jakubowski reported being able to hack the doll, despite significant steps by the manufacturer to protect customer privacy. “It’s just a matter of time until we are able to replace her servers with ours, and have her say anything we want,” he said.
*
In his analysis of the automobile industry in the 1960s, Ralph Nader argued that secrecy was one of the policies most inimical to the improvement of car safety. “Not only does this industry secrecy impede the search for knowledge to save lives … but it shields the automobile makers from being called to account for what they are doing or not doing.” We can see a similar force at work today when algorithms are used as a substitute for human decision-making without proper transparency or accountability. Proprietary algorithms used by the government are often kept secret for security reasons, or by the manufacturing companies for commercial reasons, so they can charge for the use of their product. This lacuna of transparency and accountability undermines equality of opportunity and conceals inequality in outcomes. We need to force “black box“ algorithms open.
Algorithms designed to analyze DNA evidence for use in criminal cases is a good case in point. DNA evidence is becoming a highly complex field, as testing for the presence of DNA can occur with increasingly tiny samples. As a result, samples almost always display DNA mixing, where multiple matches can be made to individuals. This can happen with objects touched by different people over hours or even days. The extent to which each person contributes to the mix depends on many factors, such as the rate at which they shed DNA material, rather than simply the order in which they touched it. Such complex samples are becoming difficult to analyze, especially by human lab technicians, and have produced results that were later discredited. In such a climate, government officials are increasingly relying on computerized processes to analyze these samples, processes often provided by private companies.
Without transparency over how these programs work, there is a gross potential for injustice. DNA evidence is compelling to juries, and a computerized process for generating results only strengthens this tendency. In New York City, some defense lawyers objected to the use of such evidence on grounds that the method was not accepted as reliable by the scientific community. The defense lawyers were denied access to the code for the program and so were unable to ascertain the logical inputs that went into the algorithm. With the help of science and math interns and forensic experts, legal aid lawyers managed to reverse-engineer the software. This was a monumental effort from public defenders, and one that ultimately bore fruit. After listening to extensive expert testimony, the judge decided that such evidence was unreliable, hence inadmissible. But this ruling was not the end for computerized DNA testing; it is still used as evidence in jurisdictions across the United States.
If public decisions are made about a person — especially if they involve a person’s liberty — there should be a right to know how this decision was reached. We no longer convict people in secretive courts, without laws of evidence, because it is rightly considered unfair. Lawyers subject expert testimony to rigorous cross-examination, and are careful to assess whether the credentials of the witness are sufficient to support the conclusions they draw. Justice must be seen to be done. In this context, DNA evidence produced by black box algorithms can be highly influential and also scientifically flawed. A more transparent process for building these algorithms is essential to protect against faulty logic working its way into our system of justice. Computer programs ought to be treated like expert witnesses. We ought to subject a program’s assumptions to a similar level of scrutiny, not treat it like an inert provider of objectively determined truth.
There is no reason why such programs could not be developed by a public authority or using public money, or could not be subject to an audit by such a body. There could be reporting guidelines, a certification process for determining whether programs produce biased outcomes, or any number of other regulatory regimes. LRMix Studio offers an alternative: it is an open source software product that interprets complex forensic DNA profiles. Similar open source tools have also been developed for matching samples to DNA databases in a way that reduces the risk of false positives and negatives. To reach the standard of reliability for acceptance by the scientific community, especially on an ongoing basis, this kind of transparency is essential.
There is a danger, of course, that making these formulas transparent gives people the opportunity to subvert them. Dedicated criminals might learn how to avoid shedding DNA on crime scenes, for example. But these problems are hardly novel, they do not foreclose other methods of evidence collection, and they do not form a good enough excuse to entrench a world of baked-in bias. The privilege against self-incrimination and the right to legal counsel are accepted as vital to a functioning criminal justice system. They both also happen to make it easier for guilty people to walk free. Nonetheless, we accept them as necessary for the proper administration of justice. The same ought to be true for computer programs used to aid decision-making in the criminal justice system.
Much like how we expect transparency over budgetary decisions or public resource allocation, algorithms used for public decision making purposes should be available for scrutiny to ensure that the logic and data inputs are fair. There are growing calls for governments to reject the use of black box algorithms in public decision-making processes and open up all code to public scrutiny. These are good starting points and can be part of a longer-term objective to apply this kind of scrutiny beyond just public bodies.
Private companies are now at the center of research into the sophisticated algorithms that go into machine learning. The academy is no longer able to compete with the vast resources that have been centralized by the data boom in companies like Google, Facebook and Amazon. This is particularly true as the technology industry invests heavily in machine learning, sucking its skilled professionals into private enterprise, largely excluding the public from the benefits of these developments. According to the vice president of Microsoft Research, Peter Lee, the cost of acquiring a top researcher in 2017 was around the same as the cost of signing a quarterback in the NFL. As Wired observed, “since then, the market for talent has only gotten hotter … big players are now buying up AI startups before they get off the ground.”
The basics of this philosophical field/figuring out how to teach machines to imitate human intelligence — will eventually become more readily available to other organizations over time as they are deployed. But there are further ways in which the development of this technology is held hostage by a small collection of companies. This kind of research requires extensive computing power and large amounts of data, both of which tend to be concentrated in large tech platforms.
The logical inputs that inform machine learning are deeply complex, to the point that even individual engineers might struggle to explain how a machine comes up with a particular response. In such circumstances, rigorous testing and standards are essential to catch problems before they are unleashed on the public. While there have been important recent initiatives oriented toward self-regulation of the industry, these are not sufficient. We need intervention by public, democratically accountable authorities. We need to start thinking about how we can infuse machine learning, like all fields of technological development, with principles of justice and fairness and make them accessible so that the benefits of these advances can be shared. We need to understand how concentrations of power are standing in the way of this objective.
In Dowie’s documentation of the Pinto disaster, he discusses all the ways in which Ford and the relevant regulator, National Highway Traffic Safety (NHTS), negotiated the drafting and implementation of new standards for the industry. Dowie outlines how Ford was able to delay, for years, the implementation of standards that it would struggle to meet without a costly redesign. The NHTS did eventually find that the Pinto contained a safety defect, and this prompted its recall by Ford. But subsequent analysis of the scandal reveals how the safety defect found by the NHTS was the result of manipulation of the standard testing. Among other things, the NHTS increased the speed of crash tests; it used a different kind of vehicle as the “bullet car” in the collision with the Pinto, weighted to make maximum contact with the fuel tank; and it ensured that the headlights were switched on to provide a possible source of ignition. The NHTS justified these changes on the basis that they would bring the tests closer to real-world scenarios. But the clear effect of this redesign was that the car would fail. The Pinto burst into flames upon impact.
The implication was that these modifications to the standard testing process were done in response to public outrage, generated by Dowie’s article and the lawsuits. Ford was held to standards that other companies were not-standards it had no way of knowing that it was required to meet. Ford has never admitted that it did anything wrong.
Yet the lesson is this: as we learn more about an industry and what is possible technologically, we need to update our expectations in terms of safety and accountability. We need to organize activists, lawyers and journalists to highlight the human consequences of badly designed technology, and force the industry to adapt to design culture that values safety and works to mitigate bias. We need to demand that governments intervene in this industry to establish publicly determined standards and methods for holding companies accountable when they are breached. The standards must be constantly updated and responsive to changing circumstances as we learn more about the problems and experiment with solutions.
*
Why shouldn’t we have crash testing for artificial intelligence? Or a certification process for machine learning? Why shouldn’t we have a panel of experts who are required to remain independent from industry, who can offer guidance on testing and addressing bias before code is shipped or security risks before a product is sold, and investigate instances when they managed to slip through the net? We need to demand broader and more representative samples of people that beta-test new technologies, to ensure designers get feedback from outside the average pool of users. We need to incorporate proper feedback loops and meaningful appeal channels for people who are subject to automated decision-making, so that mistakes are not left for users to resolve individually. We must find ways to avoid privileging existing data over the relatively unknown, to avoid importing biases from available data sets and underestimating the limits of our knowledge. We need more humans supervising automated decisions, and we must stop unthinkingly using the latter as a replacement for the former. We need to develop public guidelines about best-practice algorithms and design processes, and empower agencies to monitor standards in ways that are accountable.
“The regulation of the automobile must go through three stages,” wrote Nader. “The stage of public awareness and demand for action, the stage of legislation, and the stage of continuing administration.” We ought to apply a similar approach to technology capitalism today, in which we scrutinize the industry, rally around demands for change, and put in place processes for ongoing accountability. Such regulatory processes are hardly perfect; they can be cumbersome, subject to industry capture and misdirection. But that is equally true for any number of industries that are vital to our health, such as food safety monitoring, and the regulation of medical products, and the automotive industry. Algorithms, technological devices and artificial intelligence, when badly designed, expose us to risks as much as an unhygienic meal or faulty pacemaker. If used by government, they might even compromise administrative processes that govern our social security or judicial decisions about our personal liberty. We need to think about them as products that are designed and can be modified, and reject the arguments put forward by the tech elites to deny responsibility for their impact and blame users instead.
View Original Article: https://longreads.com/2019/05/14/technology-is-as-biased-as-its-makers/